How many Cult members have tried eat.fit?
What’s the cross-sell between cult.fit and care.fit?
What is the average number of meals consumed per customer per month?
Who are the promoters and detractors of cure.fit?
What % of Cult members with an active membership in Pune have daily attendance more than the national average?
These questions don’t ring a bell, do they?
But at Cure.Fit, these are a few of the many metrics we track as a business so that we can offer valuable & relevant health products and services to you. These are the data points that drive our decision-making and it is this approach that has helped us reach the “Data savvy maturity” level in Dell Data Maturity Model (DDMM).
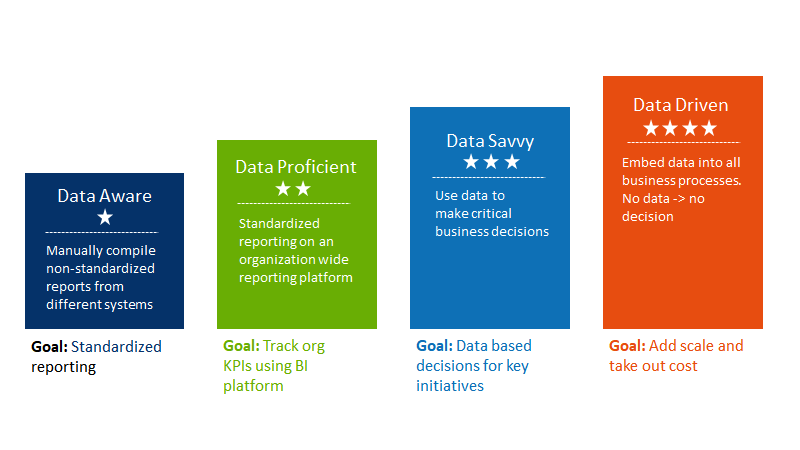
Thanks to our in-house team of expert engineers, we achieve our complex goals keeping the following objectives in mind.
- Single Source of Truth: To have a single centralised data warehouse and a consistent view of data
- Ease of KPI Definition and Computation: To provide robust support for data modelling, KPI definition building and periodic computation
- Real-Time Data Ingestion: To help answer the questions as events unfold (e.g. FitStart Sale)
- Self-Serve Query System: To enable users to run customised data queries and perform analytics.
- Uniform Reporting Format: To have a uniform and templatized report outputs to easily comprehend the data.
Data is crucial to make smart decisions. 3 years back, we at Cure.Fit started out by collecting huge data sets generated from offline centres, the mobile application, Google Analytics, CleverTap, Freshdesk, Mixpanel, etc. However, answering these questions took time and for most of the teams, the data remained inaccessible. To address these issues that plagued smooth operations, our team developed smarter solutions with the help of Hevo ETL tool to ease the workflow.
Currently, there are two types of data ingestion systems:
- Traditional ETL/ELT pipelines that fetch transactional data periodically and store them in the Data warehouse
- S3-based data lake application event streams
Current Systems
Our present analytics systems can be broadly classified into 3 sections:

ETL Pipelines:
At Cure.Fit, we ingest over 1.5 billion events per month with over 100 active pipelines and 10+ unique sources.
We leverage S3 data export and AWS Lambda to pull application events data. The rest of the sources are integrated with the help of Hevo ETL tool. Hevo has worked well for us till date and provided us with out-of-the-box features such as integration with multiple sources, python interactive transformation layers etc.
Data Warehouse and Data Lake:
We use two types of Data Warehouses to solve the different use cases. While we started out with AWS Redshift, we soon recognized the need for another DWH that will help take off selective queries from Redshift. Redshift worked perfectly during the initial days when users were less. However, with the increase in user base, we found that concurrency became a bottleneck.
So we adopted Postgres to power near real time use cases storing only 15 days of data with a sync frequency of 15 mins and shifted redshift to 1 day data sync. This allowed us to offload users from Redshift who didn’t have the need to access historical data.
As it can be seen, the volume of cold data started to increase in Redshift. This called for archiving data to S3 to save the storage cost. But, the challenge here was how do we make this historical data queryable from S3 as and when required. Redshift Spectrum came to our aid here, helping us to query S3 data directly through Redshift based on a pay per scan costing model.
Below listed are a few of the common pitfalls/ suggestions, you should take into consideration when setting up Redshift + Spectrum cluster.
- Pick the Distkey and Sortkey wisely as they can make a huge difference when it comes to the performance of queries
- Classify the users who use Redshift in categories and set up the WLM + QMR rules accordingly
- Redshift cluster concurrency can prove to be very useful. So, don’t miss out harnessing the 1 hour/day free scaling at peak usage hours
- Encoding can save a lot of space. So, never leave it on default
- Always partition and compress the data in S3 before using Spectrum as a miss can lead to an elevation in the costs
More details about these points will be discussed in the Cure.Fit Redshift Technical Blog.
Data Visualisation, Dashboards & Reporting
At Cure.Fit, Metabase is the single point of contact for all data access needs of business users, the only prerequisite being basic SQL knowledge. Holding an active user base of around 800+ individuals who use Metabase, on average, we have found that 450 users use it daily for analytics. Furthermore, every single day over 200,000 queries are run on metabase.
The top features of Metabase include attractive UI, alarms, slack integration, graphs, and UI-based query interaction for non-SQL users. Simply put, Metabase is serving us pretty well with our current use cases.
Here are a few examples of popular dashboards we refer to on Metabase:
- Cure.Fit Product Ratings Dashboard
- User Churn Dashboards
- User Acquisition Cohorts
At Cure.Fit, we have also altered Metabase to serve a few additional use cases such as:
- Support Multi-Time Zone Queries
- Mongo Query Support
We also send daily emails to users who are subscribed to their respective KPI reports. This helps them stay on top of their business metrics and helps business users get their day started.
What The Future Holds For cure.fit
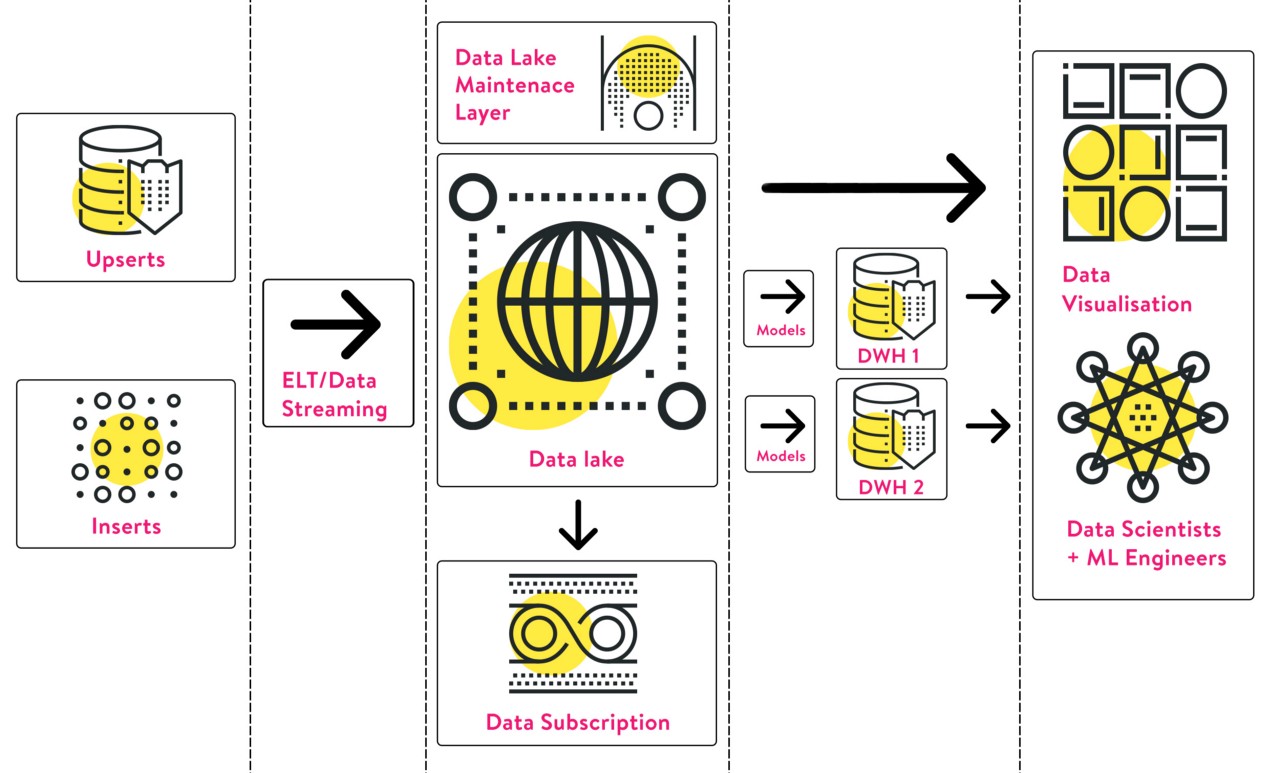
Back in Jan 2019, we were consuming over 350 million events every month. However, today, we have grown by 5X, and our 110 cult centres have grown to 250+ centres which is a 2X growth. Furthermore, our verified user base has also grown by 4X. With the increase in user base, the volume of data and the complexity surrounding it also increased.
Back then, we had also started ingesting IoT data which in itself is pretty huge. All our achievements point towards the single direction of attaining cost-effectiveness and architecture that can hold a different variety of data together and still be easily accessible.
In short, we need a Central Data Lake with high reliability. Which means decoupling the storage and computation is the way forward.
With the amount of data we ingest, the main challenge would be to achieve our objective of a single point of truth.
Watch this space for more on how we evolve our analytics stack to meet the demands of a fast growing business.
PS: We’re hiring! If you’d like to to work with us, send in your resume to careers@curefit.com
Credits — Nilesh Sinha, Manoj Tharwani